Precision and digital medicine: transforming the patient journey and medical educationadminpmls20162021-03-05T04:40:15-05:00
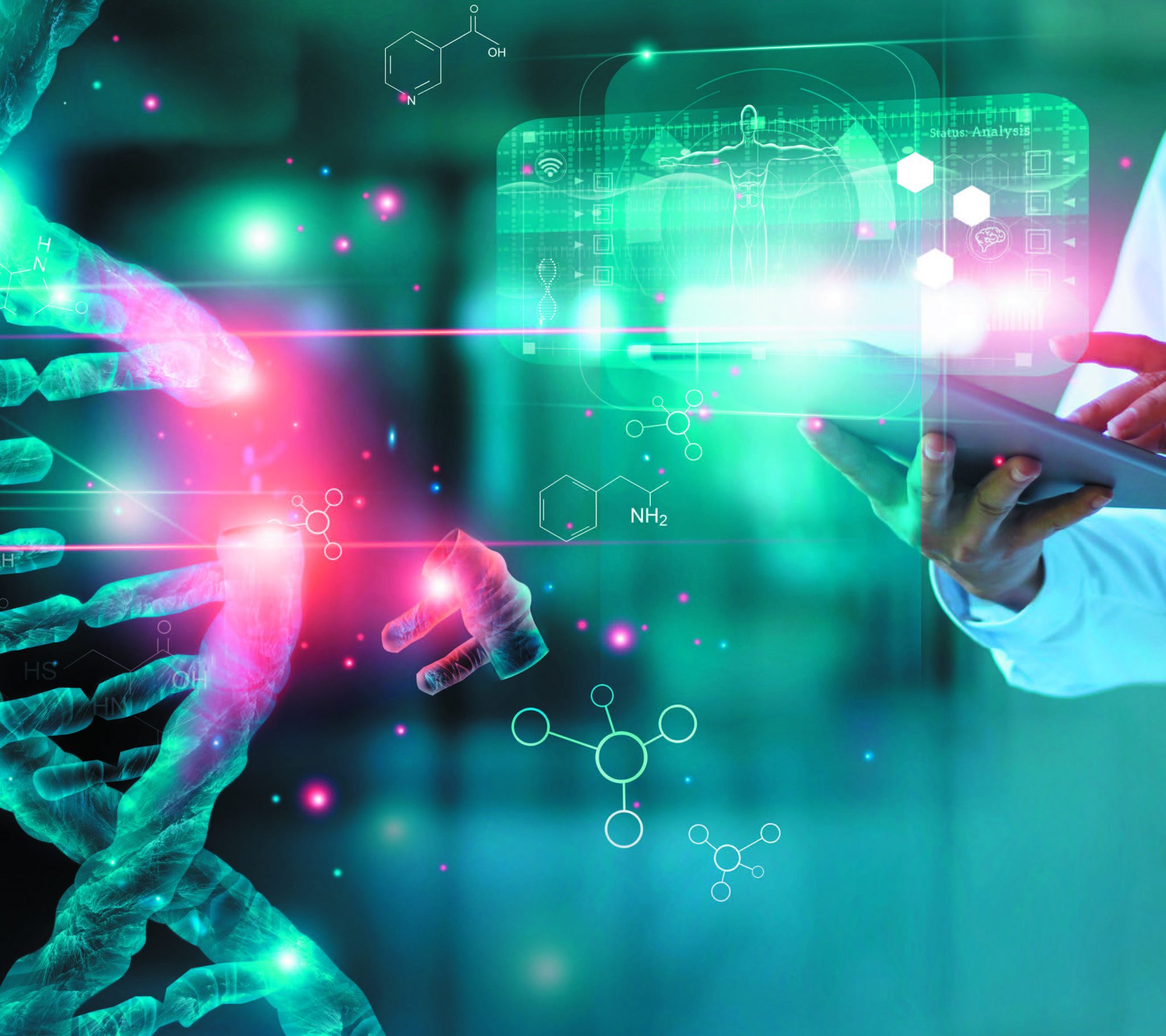
Precision and digital medicine: transforming the patient journey and medical education
by Bryn Roberts and Luke Roberts
Motivation
We’d like to start with a story, a story of a life transformed – a family transformed – through the application of genomics in precision diagnostics. Jessica has a rare disease that affected her early development due to a single defective gene. Her story demonstrates the power of precision diagnostic tools that can be brought to bear on understanding disease aetiology and, in a growing number of cases, lead to safe and effective precision medicine interventions. In the following, we’ll describe the patient journey as transformed by precision medicine and close with the outcome of Jessica’s journey.
Introduction
The FDA’s description of precision medicine contrasts with the long‑standing, one‑size‑fits
all approach designed for the “average patient.” The precision medicine approach attempts to use population statistics to target the right treatments to the right patient populations at the right time while reducing the overall risk of adverse effects.1 Personalized medicine is practiced by a physician based on the specifics of a single patient’s genetics, environment, lifestyle, clinical and phenotypic data, genomics, lifestyle, family history, and recent treatments with the goal of improving outcomes (safely and effectively).
A patient’s journey is uncertain from the start and is too often a twisting, tortuous route with setbacks and disappointments. A goal of precision medicine is to reduce the uncertainty of the path by using diagnostics and drugs targeted to the patient’s condition. Thanks to ongoing efforts to annotate and sequence the cancer genome, the FDA embraced the goal of reducing uncertainty by increasing precision in medicine. The FDA built the foundation of its Precision Medicine Program (now ‘‘All of US’’, https://allofus.nih.gov/) on cancer, where significant advances in treatments (e.g., for tumorigenesis or subsequent processes such as invasion or metastases) have been developed in recent years by targeting specific genetic variations. However, a personalized, or precision medicine approach is applicable not only in oncology, nor is it based solely on genetic or genomic factors. Over time, one looks to extend the value proposition to cover both individual patient cases as well as public health and societal benefits to realize overall reduced cost of healthcare and increased productivity.2
Figure 1: Overview of the patient journey showing the major stages, potential intercept points and key iterative loops.
Focusing on next generation sequencing and oncology provides a relatively narrow view of the goal of the “right‑treatment, right‑patient, right‑time” approach. The principle applies to all disease areas where deeper insights into the biology underlying an individual’s medical condition enables differential diagnosis and more targeted treatment options. Although the exact history of precision medicine is debated, examples were certainly evident by the turn of the 20th century and well established by the 1970s.3,4 The concept of precision phenotyping5 is as important as genetics in many situations, utilizing data from biochemical, physiological, multi‑omic, imaging, and behavioral measurements to provide more precise disease classification and etiological insights; these data sets can inform subsequent precision treatment options.6 To simplify, we postulate that precision medicine is, in fact, based on three underlying requirements: precision diagnostics, precision treatments, and precision monitoring of response and/or disease progression, which play out along the journey of a patient. A fourth condition, to satisfy the definition of “right‑time,” requires that these three factors should be available and effective as early as possible in the journey.
In this paper we consider the journeys of three patient archetypes and how next generation diagnostic and monitoring tools, advances in precision phenotyping and digital health, in addition to genetics and genomics, are supporting the Precision Medicine goal. Behind many of these innovations, recent progress in artificial (or augmented) intelligence (AI) reveals extraordinary opportunities to transform the patient journey, improve outcomes and reduce the cost of healthcare for society.
We will explore the potential for the patient journey to evolve from a service‑oriented to an experience‑oriented concept, based on models from the “experience economy.” In the US, this model could also result in changing from the “fee‑for‑service” model to a “fee‑for‑outcome” model, thereby leading to overall savings for the patient and healthcare system. Finally, we will explore how personalized healthcare (PHC) will redefine not only the patient journey and experience but also the role of medical practitioners and the future of medical education and training.
The Patient Journey
The patient journey has been described as “the ongoing sequence of care events which a patient follows from the point of access into the health system, continuing towards diagnosis and care, and ending in outpatient care. In reality, this journey is a cyclical, never‑ending interaction of care events with their healthcare provider”7 and has been discussed in a number of contexts, such as patient engagement and empowerment.8 Rather than considering the journey from the perspective of the healthcare system, we will expand our view to be more patientcentric; embracing topics such as risk factors that individuals and families may face, and the related steps involved in early detection of disease, during the ‘pre‑patient’ status, when manifestations of early disease are sub‑clinical. Since entry into the healthcare system triggers the state transition to ‘patient’, we may describe this pre‑patient status and ecosystem as ‘wellcare,’ in contrast to healthcare.
The high‑level stages in our journey are shown in Figure 1. The typical entry point into the healthcare system occurs once symptoms become apparent to the individual or are detected during routine health screening. With the advent of innovative precision and digital health solutions, opportunities are created to intercept and break the journey cycle, based on risk factors and/or early detection of sub‑clinical symptoms. Precise and accurate tests, supporting sensitive and specific diagnostics, enable selection of precision treatments, where available, and are being transformed by technological innovation in domains such as medical imaging, sequencing and multi‑omics.
Figure 2: Example pairings of targeted cancer medicines and their respective companion diagnostic tests.
Early detection and intervention, based on predictive analytics, have been shown to both reduce healthcare costs and improve outcomes for patients.9 Digital health solutions (DHS) offer huge potential to transform the early detection and monitoring phases of the journey, which are essentially the same activities just pre‑ and post‑diagnosis, respectively. DHS offer the ability to measure multiple symptom domains with high sensitivity and objectivity, in the real‑world setting, in real‑time, with affordable and accessible tools. The potential for individuals to become their own baseline control, through continuous longitudinal measurement, provides an additional degree of personalization.
After a disease has been diagnosed at a generic clinical level, often in terms of anatomy, pathology and function, further differentiation of the disease, based on cellular and molecular mechanisms, clarify prognosis and enable personalised precision treatment options. Many examples in our three highlighted disease areas have been investigated and continue to be active areas of research since significant unmet needs still remain for these patients.10,11,12
Established practice in oncology demonstrates interdependency between a precision (targeted) treatment and a precision diagnostic. One without the other severely curtails the potential of practicing precision medicine. Since the 1990s, the pairings of targeted medicines and their respective companion diagnostic tests have revolutionized the treatment of many cancers. Some key examples are shown in Figure 2.
Oncology is ideal for such approaches, given the underlying somatic genetic mutations that drive most cancers. However, we see similar targeting of therapies in the selection of effective antimicrobials based on the culture sensitivity of pathogens, where identifying the pathogen and presence of drug resistance genes is critical. Likewise, in diseases caused by germline genetic variants, such as Spinal Muscular Atrophy, Huntington’s Disease, and Cystic Fibrosis.
Figure 3: Overview of the oncology patient journey showing examples of components that enable Precision Medicine.
Figure 4: Overview of the cardiovascular patient journey showing examples of components that enable Precision Medicine.
This precision medicine approach breaks down when we lack one of the precision partners, either the precision diagnostic or the precision therapy. Such cases are the target of ongoing research and development efforts with the potential to transform the patient experience and the healthcare system with novel technologies and innovative solutions. We will look at a few such examples in the following sections.
Transformation driven by innovation in precision and digital medicine
In this section, we take a closer look at three archetypical patient journeys and examples of how innovation in precision and digital medicine are transforming both the experience of patients and healthcare professionals alike. The technologies at play include next generation diagnostic and monitoring tools, advances in precision phenotyping and digital health, in addition to genetics, genomics, and artificial or augmented intelligence (AI).
1) Oncology Detection, identification, diagnosis, monitoring
As discussed, precision medicine is already well established in the diagnosis and treatment of cancer, so what’s new in this journey? We see the enhancement of existing approaches, such as precision cytology using multiple HPV biomarkers for the prevention of cervical cancer and sequencing circulating tumour DNA (ctDNA) from blood, rather than relying on tissue biopsies.13 The potential benefits of ctDNA screening include detection of tumours of multiple types and locations simultaneously, detection of minimal residual disease after surgery, reduced need for patients to undergo inconvenient and unpleasant invasive procedures, and lower costs. It may enable earlier detection and better treatment monitoring, as sequential blood samples can be taken with relative ease. Additional clinical benefits, beyond early detection of the initial tumour presence, result from early detection of tumour recurrence and the emergence of resistance on‑treatment or, in the longer‑term, post‑treatment.
AI‑enabled image analysis is impacting a couple of phases and shows potential in use‑cases such as melanoma detection from dermatological images.14 In digital pathology and radiology, augmentation has enhanced tumour detection and led to more precise classification of tumour features.15,16 In the case of melanoma detection, we already see the emergence of several smartphone apps for early detection by self‑screening of lesions using either AI‑based image analysis or image transmission to remote expert readers.17 Despite the potential value (once sufficiently robust), these apps currently pose risks of false‑negative and false‑positive results leading to potential delayed action and unnecessary anxiety, respectively. Some apps, such as SkinVision, claim that they already significantly outperform primary care physicians. A further concern is self‑diagnosis; as the saying goes, “one who diagnoses one’s self has a fool for a patient’’.
Recent experience with cancer patients using digital applications during their monitoring phase, such as patient‑reported outcomes (PROs) for symptom tracking, have reported improved outcomes in both progression‑free and overall survival.18,19 It is assumed that these improvements result from more personalised care, mainly due to earlier detection of relapse and treatment‑induced side‑effects, resulting in more timely and effective follow‑up interventions. More studies are required to validate the exact mechanisms behind the observed benefits.
Figure 5: Overview of the neurology patient journey showing examples of components that enable Precision Medicine.
Interventions, treatments, therapies
Exploitation of the novel biology of tumours and innovations in bioengineering offer new therapeutic modalities and supporting tools, which enable personalisation and high precision interventions. They are currently expensive and difficult to scale but efforts are ongoing to address these challenges. Examples in practice and clinical development include engineered cell therapies, neo‑antigen vaccines, and personalized multicellular ex‑vivo organoid screening.
Adaptive therapy seeks to exploit the heterogeneity of cellular clones within a tumour, with their respective resistance adaptions and survival deficits, to select appropriate treatments and regimes to avoid enabling dangerous resistant clones to outgrow drug‑sensitive cells.20 Precision adaptive therapy may eventually become possible as testing technologies, such as single‑cell and ctDNA sequencing, enable precise and frequent monitoring of tumour cell composition. Manipulation of the gut microbiome has been hypothesized to influence outcomes with cancer immunotherapy, via influence on the inflammatory response of the host immune system, providing yet another potential avenue for personalized and precision medicine in oncology.21
Thousands of clinical trials are ongoing in oncology, many of which are testing novel treatments, indications, or therapeutic regimens (e.g., combinations of radio‑, chemo‑, or immuno‑therapy). Providing cancer patients with information about trials for which they may be eligible for enrollment expands their options in the treatment phase of their journey.22 To match patients with the trials that have the potential to meet their expectations requires a deep understanding of their disease,10 general health, outcome expectations,23 and preferences. We believe this constitutes an important option for patients’ experience of precise and personalized care.
2) Cardiovascular
Cardiovascular disease remains the number one cause of death world‑wide,24 so the potential benefits of improvements are vast – medically, socially and financially. Deep phenotyping using classical clinical and multi‑omics data is clearly leading the emerging role of precision medicine in cardiovascular disease,25 with the digital health revolution promising novel opportunities. Recent examples include the introduction of digital and AI‑enabled tools, which have made a significant impact during the journey of cardiovascular patients. With scaling, these tools have the potential to create extraordinary value in terms of outcomes, healthcare costs, and patient experience.
The most striking examples of ensuring the right patient receives the right treatment at the right time are in the areas of early detection, diagnosis (including triaging patients entering the healthcare system as acute or emergency cases), and patient monitoring. An example of the latter is daily patient self‑monitoring for occurrence of atrial fibrillation (AF) using a simple smartphone or smartwatch app.26 These apps not only increase patient awareness and engagement but, more importantly, empower subjects to consult medical professionals at earlier timepoints.
More elaborate AI algorithms are enabled in self‑use devices with increased functionality and complexity, such as the Cardisio,27,28 which tests for coronary heart disease (CHD) and heart attack risk, claiming to detect heart hypoperfusions with high sensitivity (95%) (but modest specificity (75%)). We foresee developers of these types of devices improving their algorithms in the future, increasing sensitivity, specificity, and the types of cardio‑pathologies covered. They may also be used on-treatment to optimize dosing of chronic treatments or for confirmation of an episode when acute therapies have been prescribed, such as Flecainide, or “pill in the pocket”, for cardioversion in paroxysmal AF.
A particularly interesting example is for AF and CHD, especially given the high and rising incidence of these conditions. Diagnosis at early stage or when asymptomatic29,30 could aid avoidance of heart attacks, strokes and other serious complications, as well as interventions prior to tissue damage in certain circumstances (e.g., monitoring for ischemia that may lead to heart or brain damage). The traditional ischemic stroke treatment of thrombolysis has been offered to patients based on timing criteria, often only being given when patients present within 4.5h of symptom onset. Advances in imaging technology are enabling a personalised eligibility approach to medical thrombolysis, determining both effectiveness and safety factors more precisely and objectively. The ischemic core and penumbra can be visualised and quantified by calculating the mismatch of diffusion-weighted imaging and perfusion-weighted imaging. Therefore, enabling eligibility for thrombolysis based on the biological timeline of ischemia rather than reported time from symptom onset. This technology-guided decision-making enables effective treatment to be given to those who would have previously not qualified and avoided in those where the objective biological timeline indicates potential safety risks.31,32,33
Effective prioritization and triage of patients entering the healthcare system as emergency cases are essential for appropriate and timely treatment. In the case of cardiac arrest, a promising new use of AI was recently reported, in which emergency call conversations were analyzed using a machine learning framework. The resulting AI model outperformed medical dispatchers in identifying out-of-hospital cardiac arrests in emergency phone calls, leading proponents to suggest that AI programs may aid in decision support for emergency medical dispatching.34 The benefits may include directing calls for faster response to the most critical patients and improved resource management in the emergency care system.
Once patients are in the care of paramedics, in primary care centers, or in hospitals, rapid cost-effective diagnosis is constrained by the availability of specialized equipment and expertise. Faster diagnosis where availability of equipment and cardiology expertise are limited will enable earlier interventions that avoid significant cost and patient risk. Innovative tools that mitigate these limitations and support precision medicine for cardiac patients include Eko Health, a company offering machine learning-based classification of abnormal heart sounds using handheld wireless digital phonocardiography,35 thereby enabling detection of conditions such as structural cardiac pathologies and device thrombosis.
Beyond novel digital solutions, innovations in genetics and classical molecular biomarkers have led to advances in precision medicine such as the introduction of the ABC bleeding risk score in 2016, which combines risk factors from Age, Biomarkers from blood, and Clinical history in support of objective decisions for anticoagulant therapy.36 In fact, a compelling case can be made for more integrative approaches that systematically combine comprehensive data sets (e.g., from family and medical histories, genetics and genomics, molecular and functional biomarkers, imaging, electrophysiology) with AI tools to tailor precise and personalized well-care and healthcare plans for individuals.37
Figure 6: A comparison of Service- verses
Experience-based models, adapted from HBR48
3) Neurology
Despite the increasing number of diagnosed cases of neurodegenerative pathologies, neurology is perhaps the disease area with the least developed precision medicine landscape. Advancements await further understanding of the underlying etiology and disease mechanisms, more robust genetic associations outside of rare and familial conditions, and mapping clinical observation and data to the complex and variable nature of the resulting phenotypes. The absence of effective disease modifying therapies in major indications such as Alzheimer’s Disease (AD) and Parkinson’s Disease (PD) presently limits precision medicine, constraining its application to optimizing symptom control and disease management. Valuable opportunities to improve the patient and caregiver experience are available in traditional care pathways by using existing therapies, lifestyle adaptions, and palliative interventions.38 Recent therapeutic breakthroughs in Multiple Sclerosis (MS), Spinal Muscular Atrophy (SMA), and Huntington’s Disease (HD) create new possibilities to include targeted therapies in the precision medicine offerings for these indications, and provide a model for what we hope will follow in the most prevalent neurodegenerative conditions.
Earlier interventions with disease modifying therapy in neuroinflammatory39 and neurodegenerative40 conditions are generally accepted as leading to better outcomes. Molecular, cognitive and anatomical changes have been observed in AD pathology cases a up to decades before symptoms becomes apparent.41 Therefore, early detection, whilst subjects appear to be completely healthy, is of increasing interest for both clinical trial enrollment and, subsequently, for timely clinical intervention.
Potential tools for practical early detection spanning several modalities, including molecular,42 imaging43 and digital tests, are expected to play a role in the detection and confirmation process. Digital monitoring tools are the most promising for low cost, high convenience, and initial detection in large populations. They offer cost-effective, sensitive, objective, and continuous, real-world multi-symptom measurement of movement, cognition, memory, mood, and sleep. The same digital biomarkers can be used later in the patient journey for precise monitoring of disease progression and/or response and adherence to treatment.44 These digital monitoring tools are not limited to degenerative neurological conditions and are being developed in conditions such as autism spectrum disorders45 and further into applications that have therapeutic value during the treatment phase, such as psychiatry.46 Regulatory authorities are grappling with enabling breakthroughs for patients and health systems through adopting digital transformation in clinical trials whilst maintaining high ethical and safety standards.47
Patient Experience
Clearly, being a patient in a healthcare system is not as trivial as experiences provided by the entertainment and hospitality industry (and not always so enjoyable)! The expansion of economic models from product- and service to experience-based has parallels in patient care, with the introduction of integrated personalized and precision medicine to the patient journey. Rather than the patient being purely a recipient of goods (e.g., medicines, prosthetics) and services (e.g., diagnoses, surgery), patients now expect and require a fully integrated journey experience.
In Figure 6, we contrast some attributes of a service‑based model with an experience‑based model. In the same way that economies follow “progression of economic value” as they move from providing goods and services to staging experiences,48 so our patient journey can follow ‘progression of medical value’ via similar principles. A staged experience still contains goods and services – it is the way that the component parts are synthesised, integrated and delivered as an orchestrated whole that creates the additional value for the recipient, our patient. This requires the provider to have an end‑to‑end design philosophy and to invest in architecture suitable for a complex system; to select the optimal component goods and services; to ensure interoperability, sustainability, and continuity; and to offer personalization in meeting the requirements and expectations of the recipient.
In most healthcare systems, this coordination and delivery of end‑to‑end patient experience is lacking, not least due to fragmentation of data and workflow systems.49 The resulting dissatisfaction and sub‑optimal outcomes could be addressed if more mature ecosystems were to be developed with more active engagement of patients.50 Implications for Medical Education and Training The rise of precision medicine as a result of technological and digital advancements provides an opportunity to improve care in a clinical setting. Paradigm shifts in medical practice can be observed repeatedly in medical history, for example, from practice based on education, experience, and intuition to evidence‑based medicine. The shift to precision medicine takes that next step in the already established clinical model by using best available evidence combined with an understanding of the patient’s measured phenotype.51 Because of the ever‑increasing complexity of the role of a doctor and the skills required of clinicians, it is vital for the implementation of precision medicine that education and support match advancements in technology. As modern medicine expands, we foresee an ever‑increasing reliance on multidisciplinary healthcare teams. Clinicians may no longer be taught all the information they will need in courses, but medical education should instead be focused on building skills for resilience to future change and the acquisition of new knowledge; communication, data interpretation, and research skills are vital. A trend not only seen in medicine is the need to change the education paradigm from “know it all” to “learn it all” in the current climate of knowledge and change.52,53
While doctors do not need to become fully qualified data scientists, they will quickly find a rudimentary understanding of data, genetics, and physiology is key to future needs.54 In light of the anticipated growth of pharmacogenomics (and eventually pharmaco‑omics), the pedagogical emphasis needs to be on skills for practice within teams of experts and effective access to knowledge.55 The advances in precision medicine will also require changes to team composition, and the integration of more data scientists and clinical geneticists.
Precision medicine is currently being practiced at specialist levels of care, and is therefore not applicable to a lot of junior clinicians and undergraduates in their current roles. Principles need to be introduced for those who go onto these specialties. Looking forward, almost all aspects of medical practice may be infiltrated by the personalized approach, as diagnostic tools become more precise, with reliable evidence for targeted therapy.
One example of an emerging tool that facilitates precision medicine is Point of Care Ultrasound (POCUS). POCUS allows for high fidelity imaging of key structures such as the heart and areas where blood may collect following trauma. POCUS is an alternative to the traditional stethoscope, and allows doctors to treat patients more precisely, based on their confirmed pathology in an emergency setting. Although POCUS facilitates more precise diagnosis of acute pathology, it requires doctors to be trained in the interpretation of ultrasound, for which there is limited capacity.56 Digital tools could allow assisted diagnosis through AI recognition of anatomical structures and pathological abnormalities superimposed using augmented reality. Other imaging and surgical fields (e.g., laparoscopic surgery) would benefit from augmented reality assistance in the identification of anatomy.57,58
Undergraduate medical education is changing to accommodate the transition to precision medicine. A number of medical schools have acknowledged the need to update their curricula, and some have already implemented additional modules in precision medicine. There are now a number of postgraduate qualifications available worldwide for further study in precision medicine, supplying the need for expert clinicians in this field.59 As the landscape of clinical medicine continues to evolve, a natural integration of these topics into standard undergraduate medical education should be seen, along with further development of specific post‑graduate training opportunities.
In Conclusion
Here in 2020 we stand at the beginning of a new decade, one promising a medical revolution. Despite the current COVID‑19 pandemic, we look forward to the new decade with great anticipation – with an expectation that patients’ journeys will be transformed. In this article we have merely scratched the surface with a few examples of innovations that will contribute. However, beyond breakthroughs in genomics, digital, multi-omics, synthetic biology, AI, and the like, we need to transform the way we train and sustain medical professionals and rethink the role of the patient (or person) in the interacting ecosystems of wellcare and healthcare.
In closing, we return to Jessica’s Story. Through the work of the Great Ormond Street Hospital, supported by Genomics England, Jessica was diagnosed with GLUT1 Deficiency Syndrome, a phenotype resulting from defective Glucose Transporter Type 1, caused by a de novo deletion in one copy of her SCL2A1 gene. Since diagnosis, Jessica’s diet has been modified to avoid glucose with high expectations that her symptoms and development will improve. Although diagnosed as a young child, earlier discovery of Jessica’s condition would have avoided years of futile testing, anxiety and ongoing damage, reminding us of the importance of early detection – preferably at birth in conditions such as this. Jessica’s “right treatment, right patient, right time” precision medicine intervention is not based on a state-of-the-art therapeutic technology, the transformation of her journey is based on a state-of-the-art diagnostic technology – whole genome sequencing. As a result, her parents feel informed and newly empowered to support Jessica in her journey ahead and understand the implications for the future of their family.60,61
Bryn Roberts – Bryn has a PhD in Pharmacology and background in Data Science. He joined Roche in Basel in 2006 and, in his current role as Global Head of Operations & Informatics for Pharma Research and Early Development, Bryn’s accountabilities include data, digital and personalized healthcare programmes, research infrastructure, automation and sustainability. Beyond Roche, Bryn is a Visiting Fellow at the University of Oxford, with interests in AI and machine learning, systems biology and scientific soft ware development. He is an Associated Faculty member with the University of Frankfurt Big Data Lab and lectures in medical informatics at the University of Applied Sciences, NW Switzerland. He is a member of several advisory boards including the Pistoia Alliance, University of Oxford Dept of Statistics and SABS Centre for Doctoral Training, the Microsoft Research/University of Trento Center for Computational and Systems Biology, and RoX Health.
Luke Roberts – Luke is in his fi nal year of study for a degree in medicine and surgery from the university of Bristol, UK. He has had the opportunity to engage with a large number of clinical specialties and patients whilst developing practical and academic skills. Although in the early stages of his clinical career, he has developed keen interest in innovation and translational medicine. Multiple internships working in early pharmaceutical research for targeted small molecule cancer therapeutics has given Luke scientifi c perspective for clinical medicine. Luke has continued to focus on innovative medicine including projects on CAR T-cell therapy, an audit focused on improving post-operative care, and furthering his education with an online course in entrepreneurship in emerging economies from Harvard Business School. Luke acts as a peer reviewer for the British Medical Journal and INSPIRE (Student Health Sciences Research Journal) with a focus on trauma, obstetrics, and gynecology.
References
- Precision Medicine. U.S. Food & Drug Administration. 2018; September 27. [available at: https://www.fda.gov/medical-devices/vitro-diagnostics/precision-medicine]
- Gavan S, Thompson A, Payne K. The economic case for precision medicine. Expert Rev Precis Med Drug Dev. 2018;3(1):1-9. doi:10.1080/23808993.2018.1421858
- Harold J. Harold on History | The Evolution of Personalized Medicine. Cardiology Magazine. 2018;October 19. [available at: https://www.acc.org/latest-in-cardiology/articles/2018/10/14/12/42/harold-on-history-the-evolution-of-personalized-medicine]
- Phillips C. Precision Medicine and its Imprecise History. Harvard Data Science Review. 2020;2(1). doi:10.1162/99608f92.3e85b56a
- Roberts B, Lee M, Pao W. Precision phenotyping to enable the development of breakthrough medicines. LinkedIn. 2019; September 24. [available at: https://www.linkedin.com/pulse/precision-phenotyping-enable-development-breakthrough-bryn-roberts]
- Yurkovich, J.T., Tian, Q., Price, N.D. et al. A systems approach to clinical oncology uses deep phenotyping to deliver personalized care. Nat Rev Clin Oncol 17, 183–194 (2020). doi:10.1038/s41571-019-0273-6
- Odoro. What is the Patient Journey? [available at: https://www.odoro.com/what-is-the-patient-journey]
- Bolz-Johnson M, Meek J, Hoogerbrugge N. “Patient Journeys”: improving care by patient involvement. Eur J Hum Genet. 2020;28:141–143 (2020). doi:10.1038/s41431-019-0555-6
- Chen P. Case study: Predictive analytics helped Sonic Healthcare cut costs and boost profits. HealthcareTransformers. 2020; October 6 [available at: https://healthcaretransformers.com/healthcare-business/case-study-predictive-analytics]
- Sporikova Z, Koudelakova V, Trojanec R, Hajduch M. Genetic Markers in Triple-Negative Breast Cancer. Clinical Breast Cancer. 2018;18(5):e841-e850. doi:10.1016/j.clbc.2018.07.023
- Making the connection: Targeting multiple mechanisms in heart failure. 2020. [available at: https://www.astrazeneca.com/what-science-can-do/topics/disease-understanding/making-the-connection-targeting-multiple-mechanisms-in-heart-failure.html ]
- Qian E, Huang Y. Subtyping of Parkinson’s Disease – Where Are We Up To?. Aging Dis. 2019;10(5):1130-1139. doi:10.14336/AD.2019.0112
- Chen M, Zhao H. Next-generation sequencing in liquid biopsy: cancer screening and early detection. Hum Genomics. 2019;13(1):34. doi:10.1186/s40246-019-0220-8
- Kassani S, Kassani P. A comparative study of deep learning architectures on melanoma detection. Tissue and Cell. 2019;58:76-83. doi:10.1016/j.tice.2019.04.009
- Harmon S, Tuncer S, Sanford T, Choyke P, Türkbey B. Artificial intelligence at the intersection of pathology and radiology in prostate cancer. Diagn Interv Radiol. 2019;25(3):183-188. doi:10.5152/dir.2019.19125
- Coudray N, Ocampo P, Sakellaropoulos, T. et al. Classification and mutation prediction from non–small cell lung cancer histopathology images using deep learning. Nat Med. 2018;24:1559–1567. doi.org/10.1038/s41591-018-0177-5
- Galan N. How effective are skin cancer apps for early detection? Medical News Today. 2019;August 21. [available at: https://www.medicalnewstoday.com/articles/285751]
- Denis F, Yossi S, Septans AL, Charron A, Voog E, Dupuis O, Ganem G, Pointreau Y, Letellier C. Improving Survival in Patients Treated for a Lung Cancer Using Self-Evaluated Symptoms Reported Through a Web Application. Am J Clin Oncol. 2017;40(5):464-469. doi: 10.1097/COC.0000000000000189
- Basch E, Deal AM, Dueck AC, et al. Overall Survival Results of a Trial Assessing Patient-Reported Outcomes for Symptom Monitoring During Routine Cancer Treatment. JAMA. 2017;318(2):197-198. doi:10.1001/jama.2017.7156
- Bacevic K, Noble R. Soffar A, et al. Spatial competition constrains resistance to targeted cancer therapy. Nat Commun. 2017;8(article no.1995). doi:10.1038/s41467-017-01516-1
- Lin S, Yang X, Li J, et al. Gut Microbiome as a Potential Factor for Modulating Resistance to Cancer Immunotherapy. Frontiers in Immunology. 2020(10):2989. doi:10.3389/fimmu.2019.02989
- Vignot S, Martin M, Albin N, Schurtz C, Chapel E. Facilitating access to new therapeutic options through clinical trials: the vision of a regulator to reconcile innovation and safety. Annals of Oncology . 2019;30(11):1694-1696. doi:10.1093/annonc/mdz389
- Miller F, Joffe S. Benefit in phase 1 oncology trials: therapeutic misconception or reasonable treatment option? Clin Trials. 2008;5(6):617-23. doi: 10.1177/174077450809757
- American Heart & Stroke Associations. Cardiovascular Disease: A Costly Burden For America Projections Through 2035. American Heart Association CVD Burden Report. 2017. [available at: https://healthmetrics.heart.org/wp-content/uploads/2017/10/Cardiovascular-Disease-A-Costly-Burden.pdf]
- Leopold J, Loscalzo J. Emerging Role of Precision Medicine in Cardiovascular Disease. Circ Res. 2018;122(9):1302-1315. doi:10.1161/CIRCRESAHA.117.310782
- Zaprutko T, Zaprutko J, Baszko A, et al. Feasibility of Atrial Fibrillation Screening With Mobile Health Technologies at Pharmacies. Cardiovasc Pharmacol Ther. 2020;25(2):142-151. doi: 10.1177/1074248419879089
- Erkenov T, Stankowski T, Grimmig O, Just S. Cardisiography as a novel non-invasive diagnostic tool for the detection of coronary artery disease at rest – a first prospective study of diagnostic accuracy. Pre-publication abstract. 2020. [available at: https://cardis.io/clinical-research]
- Braun T, Spiliopoulos S, Veltmana C, et al. Detection of myocardial ischemia due to clinically asymptomatic coronary artery stenosis at rest using supervised artificial intelligence-enabled vectorcardiography – A five-fold cross validation of accuracy. Journal of Electrocardiology. 2020;59:100-105. doi:10.1016/j.jelectrocard.2019.12.018
- Morillo CA, Banerjee A, Perel P, Wood D, Jouven X. Atrial fibrillation: the current epidemic. J Geriatr Cardiol. 2017;14(3):195-203. doi:10.11909/j.issn.1671-5411.2017.03.011
- Sanchis-Gomar F, Perez-Quilis C, Leischik R, Lucia A. Epidemiology of coronary heart disease and acute coronary syndrome. Ann Transl Med. 2016;4(13):256. doi:10.21037/atm.2016.06.33
- Wang D, Wang Y. Tissue window, not the time window, will guide acute stroke treatment. Stroke and Vascular Neurology. 2019;4(1):1-2. Doi: 10.1136/svn-2018-000211
- Chen F, Ni YC. Magnetic resonance diffusion-perfusion mismatch in acute ischemic stroke: An update. World Journal of Radiology. 2012;4(3):63. doi: 10.4329/wjr.v4.i3.63
- Xiang X, Cao F. Time window and “tissue window”: two approaches to assist decision-making in strokes. Journal of Neurology. 2018;266(2):283-288. doi: 10.1007/s00415-018-8933-5
- Blomberg S, Folke F, Ersbøll A, et al. Machine learning as a supportive tool to recognize cardiac arrest in emergency calls. Resuscitation. 2018;13:322-329. doi:10.1016/j.resuscitation.2019.01.015
- Attia Z, Dugan J, Maidens J. MDP178 – Prospective Analysis of Utility of Signals from an ECG-Enabled Stethoscope to Automatically Detect a Low Ejection Fraction Using Neural Network Techniques Trained from the Standard 12-Lead ECG. American Heart Association: Scientific Sessions. 2019; November 16. [available at: https://www.ekohealth.com/publications]
- Hijazi Z, Oldgren J, Lindbäck J, et al. The novel biomarker-based ABC (age, biomarkers, clinical history)-bleeding risk score for patients with atrial fibrillation: a derivation and validation study. The Lancet. 2016;387(10035):2302-2311. doi:10.1016/S0140-6736(16)00741-8
- Lam C, Hare J. Automating the fight against heart disease with artificial intelligence. HealthcareTransformers. 2020; September 29. [available at: https://healthcaretransformers.com/digital-health/artificial-intelligence-in-cardiology]
- Strafella C, Caputo V, Galota M, et al. Application of Precision Medicine in Neurodegenerative Diseases. Front. Neurol. 2018;9:701. doi:10.3389/fneur.2018.00701
- Hauser S, Kappos L, Arnold D, et al. Five years of ocrelizumab in relapsing multiple sclerosis – OPERA studies open-label extension. Neurology. 2020;95(13):e1854-e1867. doi: 10.1212/WNL.0000000000010376
- Marcos P, Martinez L, Ribeiro O, et al. Early diagnosis and treatment of Alzheimer’s disease: new definitions and challenges. Braz. J. Psychiatry. 2020;42(4):431-441. doi:10.1590/1516-4446-2019-0735
- Younes L, Albert M, Moghekar A, et al. Identifying Changepoints in Biomarkers During the Preclinical Phase of Alzheimer’s Disease. Frontiers in Aging Neuroscience. 2019;11:74. doi:10.3389/fnagi.2019.00074
- Blom ES, Giedraitis V, Zetterberg H, et al. Rapid progression from mild cognitive impairment to Alzheimer’s disease in subjects with elevated levels of tau in cerebrospinal fluid and the APOE epsilon4/epsilon4 genotype. Dement Geriatr Cogn Disord. 2009;27(5):458-464. doi:10.1159/000216841
- Young P, Estarellas M, Coomans E, et al. Imaging biomarkers in neurodegeneration: current and future practices. Alz Res Therapy. 2020;12(article no.49). doi:10.1186/s13195-020-00612-7
- Baker M, van Beek J, Gossens C. Digital health: Smartphone-based monitoring of multiple sclerosis using Floodlight. Nat Research (sponsor feature). 2019. [available at: https://media.nature.com/original/magazine-assets/d42473-019-00412-0/d42473-019-00412-0.pdf]
- Hale C. Cognoa’s AI-powered diagnostic app for autism clears clinical study, heads for FDA review. FIERCE Biotech. 2020;September 23. [available at: https://www.fiercebiotech.com/medtech/cognoa-s-ai-powered-diagnostic-app-for-autism-clears-clinical-study-heads-for-fda-review
- Cho CH, Lee HJ. Could Digital Therapeutics be a Game Changer in Psychiatry?. Psychiatry Investig. 2019;16(2):97-98. doi:10.30773/pi.2019.01.20
- Mantua V, Arango C, Balabanov P, Butlen-Ducuing F. Digital health technologies in clinical trials for central nervous system drugs: an EU regulatory perspective. Nature Reviews Drug Discovery. 2020;September 29. doi: 10.1038/d41573-020-00168-z
- Pine B, Gilmore J. Welcome to the Experience Economy. Harvard Business Review. 1998;7 [available at: https://hbr.org/1998/07/welcome-to-the-experience-economy]
- PwC Health Research Institute (HRI). Customer experience in the New Health Economy: The data cure. 2018. [available at: https://www.pwc.com/us/en/health-industries/health-research-institute/publications/pdf/pwc-hri-customer-experience-in-new-health-economy.pdf]
- Tai-Seale M, Downing N, Jones V, et al. Technology-Enabled Consumer Engagement: Promising Practices At Four Health Care Delivery Organizations. Health Affairs. 2019;38(3). doi:10.1377/hlthaff.2018.05027
- Goldberger J, Buxton A. Personalized Medicine vs Guideline-Based Medicine. JAMA. 2013;309(24):2559–2560. doi:10.1001/jama.2013.6629
- Ackoff R, Greenberg D. Turning Learning Right Side Up: Putting Education Back on Track. Pub: Pearson Prentice Hall. 2008. ISBN-13: 978-0132887632
- Bass D. Satya Nadella Talks Microsoft at Middle Age. Bloomberg Businessweek. 2018;August 4. [available at: https://www.bloomberg.com/features/2016-satya-nadella-interview-issue]
- Salari K. The dawning era of personalized medicine exposes a gap in medical education. PLoS Med. 2009;6(8):e1000138. doi:10.1371/journal.pmed.1000138
- Kohane I. Ten things we have to do to achieve precision medicine. Science 2015;349(6243):37-38. doi:10.1126/science.aab1328
- Smallwood N, Dachsel M. Point-of-care ultrasound (POCUS): unnecessary gadgetry or evidence-based medicine?. Clin Med (Lond). 2018;18(3):219-224. doi:10.7861/clinmedicine.18-3-219
- Nicolau S, Soler L, Mutter D, Marescaux J. Augmented reality in laparoscopic surgical oncology. Surgical Oncology. 2011;20(3):189-201. doi:10.1016/j.suronc.2011.07.002
- Bernhard S, Nicolau S, Soler L, Doignon C. The status of augmented reality in laparoscopic surgery as of 2016. Medical Image Analysis. 2017;37:66-90. doi:10.1016/j.media.2017.01.007
- Freeley M. Current postgraduate training programs and online courses in precision medicine. Expert Review of Molecular Diagnostics, 2020;20(6):569-574. doi: 10.1080/14737159.2020.1709826
- From 6 million to 1 – Jessica’s story. Genomics England & Great Ormond Street Hospital. [available at: https://www.genomicsengland.co.uk/understanding-genomics/jessicas-story]
- First children receive a genetic diagnosis at GOSH as part of the 100,000 Genomes Project. Great Ormond Street Hospital. 2016. [available at: https://www.gosh.nhs.uk/news/latest-press-releases/first-children-receive-genetic-diagnosis-gosh-part-100000-genomes-project]